Wave Computing was founded with the vision of delivering deep learning computers with game-changing computational performance and energy efficiency. Their objective is to enable businesses to analyze complex data in real-time with more accurate results through a fluid discovery and improvement in Deep Neural Network (DNN) development and training with our family of computers.
To achieve their goal, Wave developed a novel Dataflow Processing Unit (DPU) architecture as part of a strategy to natively support a new wave of dataflow model based deep learning frameworks such as Google’s TensorFlow and Microsoft’s CNTK.
They have a massively parallel dataflow processing architecture called the Wave Dataflow Processing Unit (DPU) for deep learning.
It is all about Patterns of Data
There are important patterns to be discovered within the immense volumes of unstructured data being collected by organizations. Images could contain pattern for particular face or particular car. Sensor data could contain patterns for financial fraud or indicators of equipment failure. Social network data could contain patterns indicating criminal behavior or new consumer purchase behaviors. Cost effectively uncovering these patterns to derive actionable insights on a timely basis is changing how we interact with computers, how businesses operate, and how governments provide better public safety and services.
Wave is building the world’s fastest and most energy effi cient family of Deep Learning Computers based on their disruptive dataflow technology. Wave’s Computers are built upon a novel Dataflow Processing Unit (DPU) architecture that is purpose-built for data intensive workloads such as DNNs:
(1) Each DPU containing tens of thousands of processing nodes and
(2) Software tools based on dataflow graphs to natively support deep learning frameworks such as TensorFlow from Google. The result is a Deep Learning Computer family that designed to power the next wave of smarter DNNs.
Rather than developing a “way too expensive” custom ASIC, Meyer said Wave Computing has leveraged the well-understood dataflow technology and designed a new processing unit that “can natively support Google TensorFlow and Microsoft CNTK.”
The company’s new chip, built using 16-nm process geometry, is in the last phase of tape out, according to Meyer. Wave Computing already claims a handful of customers. “We will support our lead customers under the early access program starting later this year,” he said. General availability of Wave’s Deep Learning Computers will be in 2017.
Wave’s Computers provide:
• Token-less dataflow with finely tuned micro-architecture for processing dataflow graphs at very high cycle rate.
• Real-time reconfigurable dataflow architecture with autonomous local control for flexibility and high memory bandwidth.
• Large, distributed DPU memory for e cient support of data reuse.
• Shared memory architecture between DPUs to enable better scalability.
• Natural dataflow mapping of dataflow computational model based deep learning framework such as TensorFlow and CNTK.
• Multi-precision arithmetic to enable accumulation at high precision while storing DNN parameters at lower precision for more e cient memory utilization.
• Designed to improve functional density and computational e fficiency with mechanisms to reduce power when processing elements in DPUs are not actively processing data.
The result is a scalable architecture with localized communication that is optimized for executing dataflow graphs Wave’s family of deep learning computers deliver more than 10x performance on DNN training and more than 100x performance on DNN inference workloads compared to any existing CPU-Accelerator based systems.
SOURCES- Wave Computing
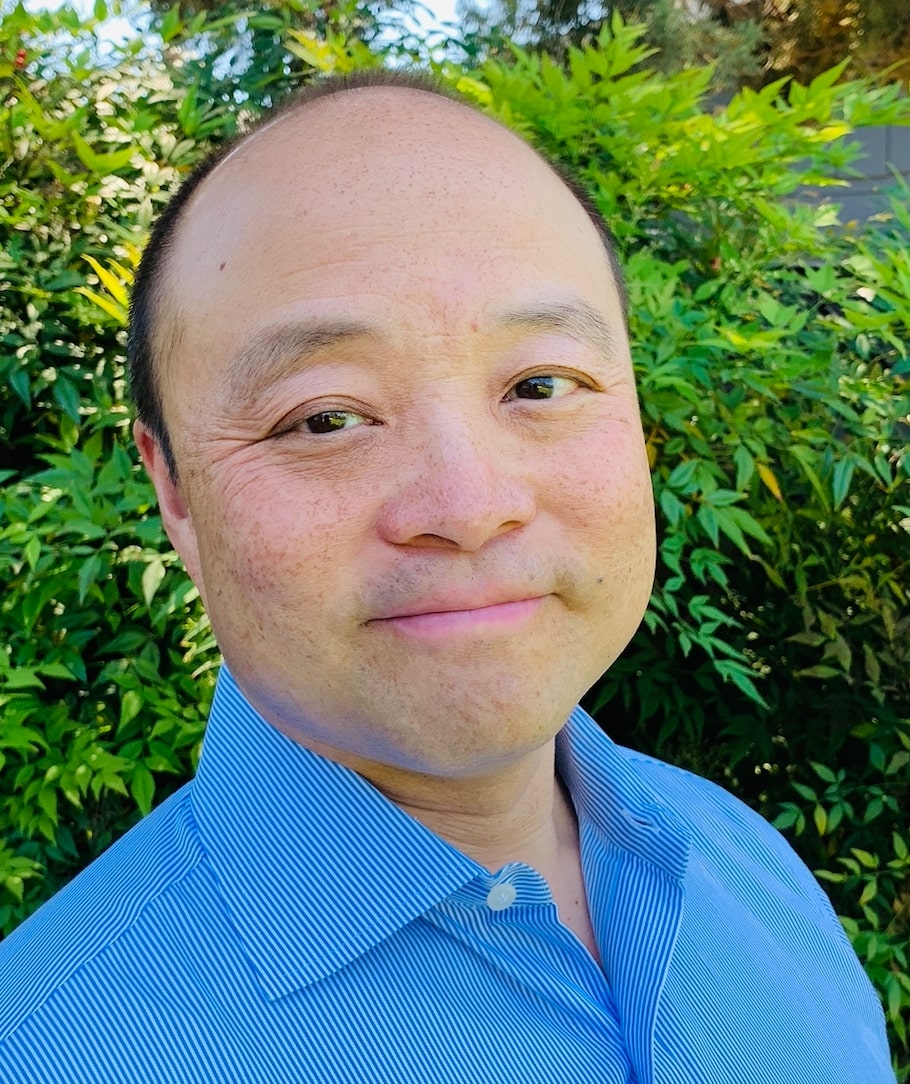
Brian Wang is a Futurist Thought Leader and a popular Science blogger with 1 million readers per month. His blog Nextbigfuture.com is ranked #1 Science News Blog. It covers many disruptive technology and trends including Space, Robotics, Artificial Intelligence, Medicine, Anti-aging Biotechnology, and Nanotechnology.
Known for identifying cutting edge technologies, he is currently a Co-Founder of a startup and fundraiser for high potential early-stage companies. He is the Head of Research for Allocations for deep technology investments and an Angel Investor at Space Angels.
A frequent speaker at corporations, he has been a TEDx speaker, a Singularity University speaker and guest at numerous interviews for radio and podcasts. He is open to public speaking and advising engagements.
Is it any good at games? World of tanks or Civ that’s where the real money is ?
As getting a decent Ai to work is a real pain