Businesses want a 100% complete AI systems that can be trained directly with just data. This is what OpenAI was able to do with ChatGPT and large language models. This is what Tesla has been able to achieve with version 12 of FSD (Full self driving).
The Tesla FSD v12 was demonstrated for nearly one hour in a live video stream by Elon Musk. A live demonstration meant that any mistakes would be shown live. There was one mistake made by the FSD v12 system in that live demo. It was wanting to make a turn based on a turn signal for different cars. The traffic like turn signal could be seen but it was for a different lane of cars. The end to end neural network FSD means that fixing this problem is done by finding examples of human drivers not proceeding in similar situations.
James Douma explains that for self driving cars and other challenging problems for neural nets, the reason that prior attempts to jump to 100% complete AI systems did not work was the training signal was too weak. The subsystems need to be improved with strong training signals and the system for getting good training video data needs to be built and refined. Once each of the components of self driving (planning, control and visual perception) are all very good then the complete system can be improved with weaker training signal data.
If this AI problem was chess, then the subsystems of the chess AI would need to get up to the level of a candidate master level before end to end training would work. This is actually not the case for chess and AI but this is an analogy for the level for self driving where the transition to end-to-end becomes beneficial.
If this AI problem was jiu-jitsu then the non-end-to end would need to progress to Purple belt level before switching to the end to end system to improve by example to black belt levels.
A significant level of mastery would be needed before the system could just look at visual examples and understand the examples to make great improvement by only looking at good examples.
James provides the diagrams of the parts of the FSD AI below and describes them in the video.
Having an end to end neural networks with a system for training it enables faster system improvement. Getting to this point required over a million good training examples. It is not just ordinary scenes or images of regular driving, it is one million curated examples of good human driving across a range of tens of thousands of situations. Reaching one million good training examples is when the FSD systems start to transition to being better than human coding by large teams of programmers.
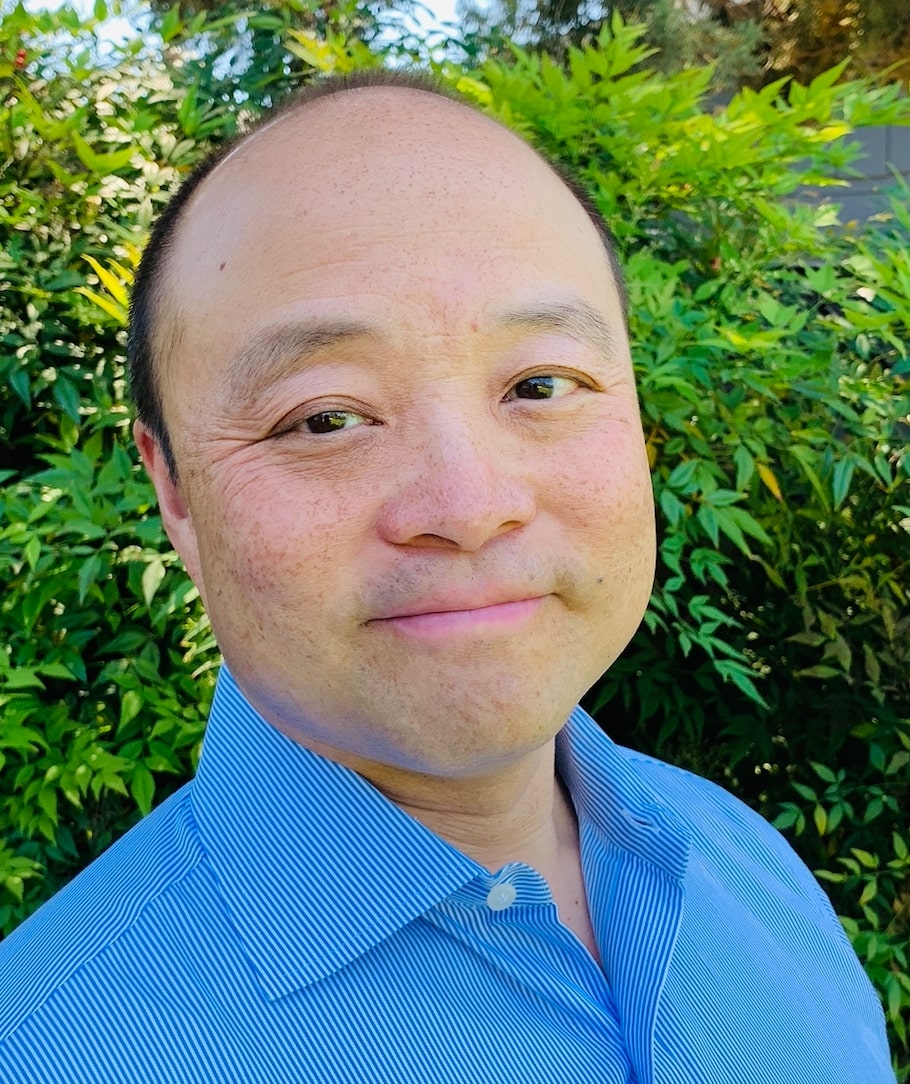
Brian Wang is a Futurist Thought Leader and a popular Science blogger with 1 million readers per month. His blog Nextbigfuture.com is ranked #1 Science News Blog. It covers many disruptive technology and trends including Space, Robotics, Artificial Intelligence, Medicine, Anti-aging Biotechnology, and Nanotechnology.
Known for identifying cutting edge technologies, he is currently a Co-Founder of a startup and fundraiser for high potential early-stage companies. He is the Head of Research for Allocations for deep technology investments and an Angel Investor at Space Angels.
A frequent speaker at corporations, he has been a TEDx speaker, a Singularity University speaker and guest at numerous interviews for radio and podcasts. He is open to public speaking and advising engagements.