Ben Goertzel – My initial reaction to reading about IBM’s “Watson” supercomputer and software was a big fat ho-hum. Watson is a fancy database lookup system. Watson is a trimph of Natural Language Processing. But while that cynical view is certainly technically accurate, I have to admit that when I actually watched Watson play Jeopardy! on TV — and beat the crap out of its human opponents — I felt some real excitement … and even some pride for the field of AI.
I found Watson’s occasional really dumb mistakes made it seem almost human. If the performance had been perfect there would have been no drama — but as it was, there was a bit of a charge in watching the computer come back from temporary defeats induced by the limitations of its AI. Even more so because I’m wholly confident that, 10 years from now, Watson’s descendants will be capable of doing the same thing without any stupid mistakes.
What does Watson Mean for AI?
But who is this impassive champion, really? A mere supercharged search engine, or a prototype robot overlord?
A lot closer to the former, for sure. Watson 2.0, if there is one, may make fewer dumb mistakes — but it’s not going to march out of the Jeopardy! TV studio and start taking over human jobs, winning Nobel Prizes, building femtofactories and spawning Singularities.
But even so, the technologies underlying Watson are likely to be part of the story when human-level and superhuman AGI robots finally do emerge.
Watson is a triumph of the branch of AI called “natural language processing” (NLP) which combines statistical analysis of text and speech with hand-crafted linguistic rules to make judgments based on the syntactic and semantic structures implicit in language. Watson is not an intelligent autonomous agent like a human being, that reads information and incorporates it into its holistic world-view and understands each piece of information in the context of it own self, its goals, and the world. Rather, it’s an NLP-based search system — a purpose-specific system that matches the syntactic and semantic structures in a question with comparable structures found in a database of documents, and in this way tries to find answers to the questions in those documents.
Although Watson is “just” an NLP-based search system, it’s still not a trivial construct. Watson doesn’t just compare query text to potential-answer text, it does some simple generalization and inference, so that it represents and matches text in a somewhat abstracted symbolic form. The technology for this sort of process has been around a long time and is widely used in academic AI projects and even a few commercial products — but, the Watson team seems to have done the detail work to get the extraction and comparison of semantic relations from certain kinds of text working extremely well. I can quite clearly envision how to make a Watson-type system based on the NLP and reasoning software currently working inside our OpenCog AI system — and I can also tell you that this would require a heck of a lot of work, and a fair bit of R&D creativity along the way.
While Watson’s use of natural language understanding and symbol manipulation technology is extremely narrowly-focused, the next similar project may be less so.
t it’s important to remember the difference between the Jeopardy! challenge and other challenges that would be more reminiscent of human-level general intelligence, such as
* Holding a wide-ranging English conversation with an intelligent human for an hour or two
* Passing the third grade, via controlling a robot body attending a regular third grade class
* Getting an online university degree, via interacting with the e-learning software (including social interactions with the other students and teachers) just as a human would do
* Creating a new scientific project and publication, in a self-directed way from start to finishWhat these other challenges have in common is that they require intelligent response to a host of situations that are unpredictable in their particulars — so they require adaptation and creative improvisation, to a degree that highly regimented AI architectures like Deep Blue or Watson will never be able to touch.
Some AI researchers believe that this sort of artificial general intelligence will eventually come out of incremental improvements to “narrow AI” systems like Deep Blue, Watson and so forth. Many of us, on the other hand, suspect that Artificial General Intelligence (AGI) is a vastly different animal (and if you want to get a dose of the latter perspective, show up at the AGI-11 conference on Google’s campus in Mountain View this August). In this AGI-focused view, technologies like those used in Watson may ultimately be part of a powerful AGI architecture, but only when harnessed within a framework specifically oriented toward autonomous, adaptive, integrative learning.
If you liked this article, please give it a quick review on ycombinator or StumbleUpon. Thanks
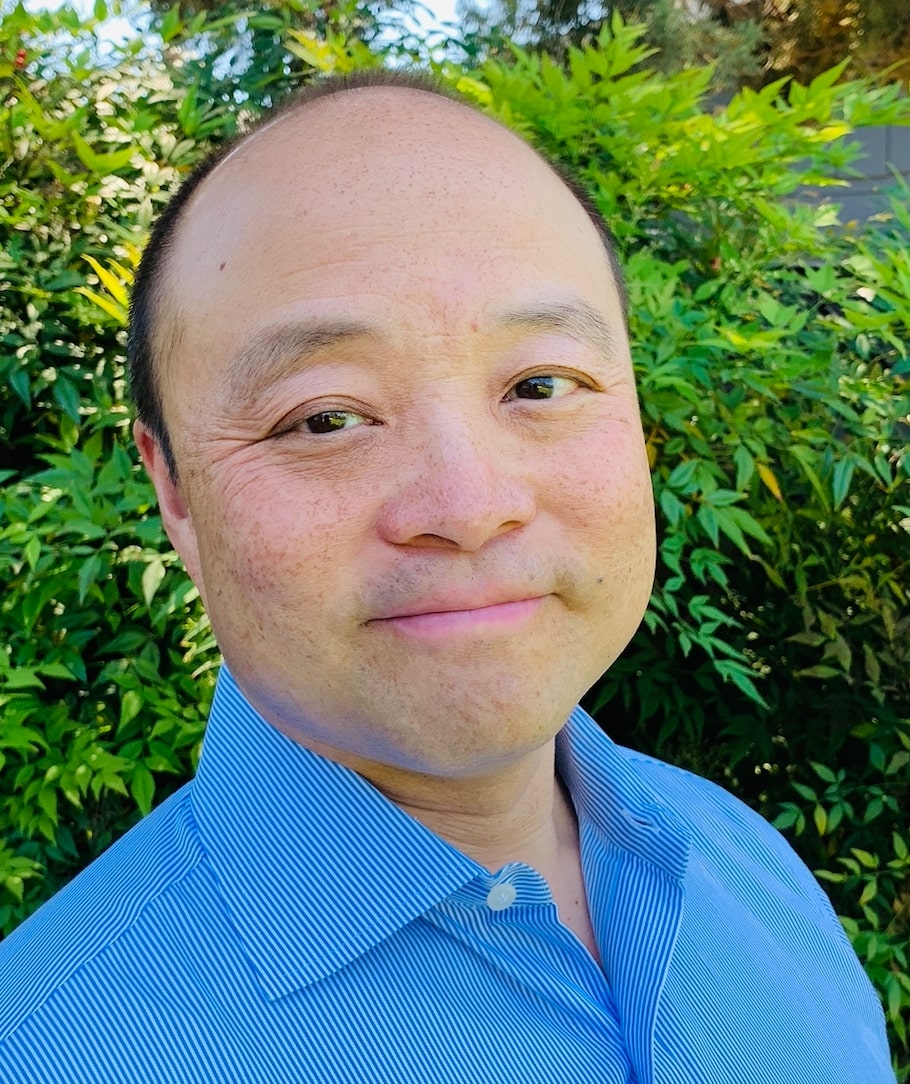
Brian Wang is a Futurist Thought Leader and a popular Science blogger with 1 million readers per month. His blog Nextbigfuture.com is ranked #1 Science News Blog. It covers many disruptive technology and trends including Space, Robotics, Artificial Intelligence, Medicine, Anti-aging Biotechnology, and Nanotechnology.
Known for identifying cutting edge technologies, he is currently a Co-Founder of a startup and fundraiser for high potential early-stage companies. He is the Head of Research for Allocations for deep technology investments and an Angel Investor at Space Angels.
A frequent speaker at corporations, he has been a TEDx speaker, a Singularity University speaker and guest at numerous interviews for radio and podcasts. He is open to public speaking and advising engagements.