DeepMind has shared the discovery of 2.2 million new crystals – equivalent to nearly 800 years’ worth of knowledge. They introduce Graph Networks for Materials Exploration (GNoME), a new deep learning tool that dramatically increases the speed and efficiency of discovery by predicting the stability of new materials.
Nature – Scaling deep learning for materials discovery
This includes 380,000 stable materials that could power future technologies. These are the promising candidates for experimental synthesis. Among these candidates are materials that have the potential to develop future transformative technologies ranging from superconductors, powering supercomputers, and next-generation batteries to boost the efficiency of electric vehicles.
They have an automatic robotic lab to make two the targets every day.
The A-Lab is an autonomous laboratory for the solid-state synthesis of inorganic powders. This platform uses computations, historical data from the literature, machine learning (ML) and active learning to plan and interpret the outcomes of experiments performed using robotics. Over 17 days of continuous operation, the A-Lab realized 41 novel compounds from a set of 58 targets including a variety of oxides and phosphates that were identified using large-scale ab initio phase-stability data from the Materials Project and Google DeepMind. Synthesis recipes were proposed by natural-language models trained on the literature and optimized using an active-learning approach grounded in thermodynamics. Analysis of the failed syntheses provides direct and actionable suggestions to improve current techniques for materials screening and synthesis design. The high success rate demonstrates the effectiveness of artificial-intelligence-driven platforms for autonomous materials discovery and motivates further integration of computations, historical knowledge and robotics.
The A-Lab integrates robotics with the use of ab initio databases, ML-driven data interpretation, synthesis heuristics learned from text-mined literature data and active learning to optimize the synthesis of novel inorganic materials in powder form. Although autonomous workflows based on liquid handling have been demonstrated in organic chemistry the A-Lab addresses the unique challenges of handling and characterizing solid inorganic powders. These often require milling to ensure good reactivity between precursors, which can have a wide range of physical properties related to differences in their density, flow behaviour, particle size, hardness and compressibility. The use of solid powders is well suited for manufacturing and technological scaleup, and the approach of the A-Lab to synthesis produces multigram sample quantities that facilitate device-level testing.
This unexpectedly high success rate (71%) for the synthesis of computationally predicted compounds was achieved by integrating robotics with:
(1) DFT-computed data to survey the energetic landscape of precursors, reaction intermediates and final products;
(2) heuristic suggestions for synthesis procedures obtained from ML models trained on text-mined synthesis data;
(3) ML interpretation of experimental data; and
(4) an active-learning algorithm that improves on failed synthesis procedures.
The study also revealed several opportunities to enhance the lab’s active-learning algorithm by addressing failures caused by slow reaction kinetics, which would enable an improved success rate of 74% with in-line solutions.
Our paper demonstrates that autonomous research agents can markedly accelerate the pace of materials research. Researchers initialized the A-Lab by proposing 58 target materials, which were successfully realized at a rate of over 2 new materials per day with minimal human intervention. Such rapid discovery points to a vast landscape of opportunities in materials synthesis and development. Although this work focused on a limited subset of all possible synthesis targets, many new candidates await evaluation. As the breadth of ab initio computations continues to grow, so will this list of novel materials.
The A-Lab demonstrates this by combining modern theory-driven and data-driven ML techniques with a modular workflow that can discover novel materials with minimal human input. Lessons learned from continuing experiments can inform both the system itself and the greater community through systematic data generation and collection. The systematic nature of the A-Lab provides a unique opportunity to answer fundamental questions about the factors that govern the synthesizability of novel materials, serving as an experimental oracle to validate predictions made on the basis of data-rich resources such as the Materials Project. In future iterations of the platform, such an oracle may be expanded to investigate factors beyond synthesizability, including microstructure and device performance. Although our current success rate for the synthesis of novel compounds is high, the remaining discrepancies between current predictions and their experimental outcomes is a crucial signal required to improve our understanding of materials synthesis.
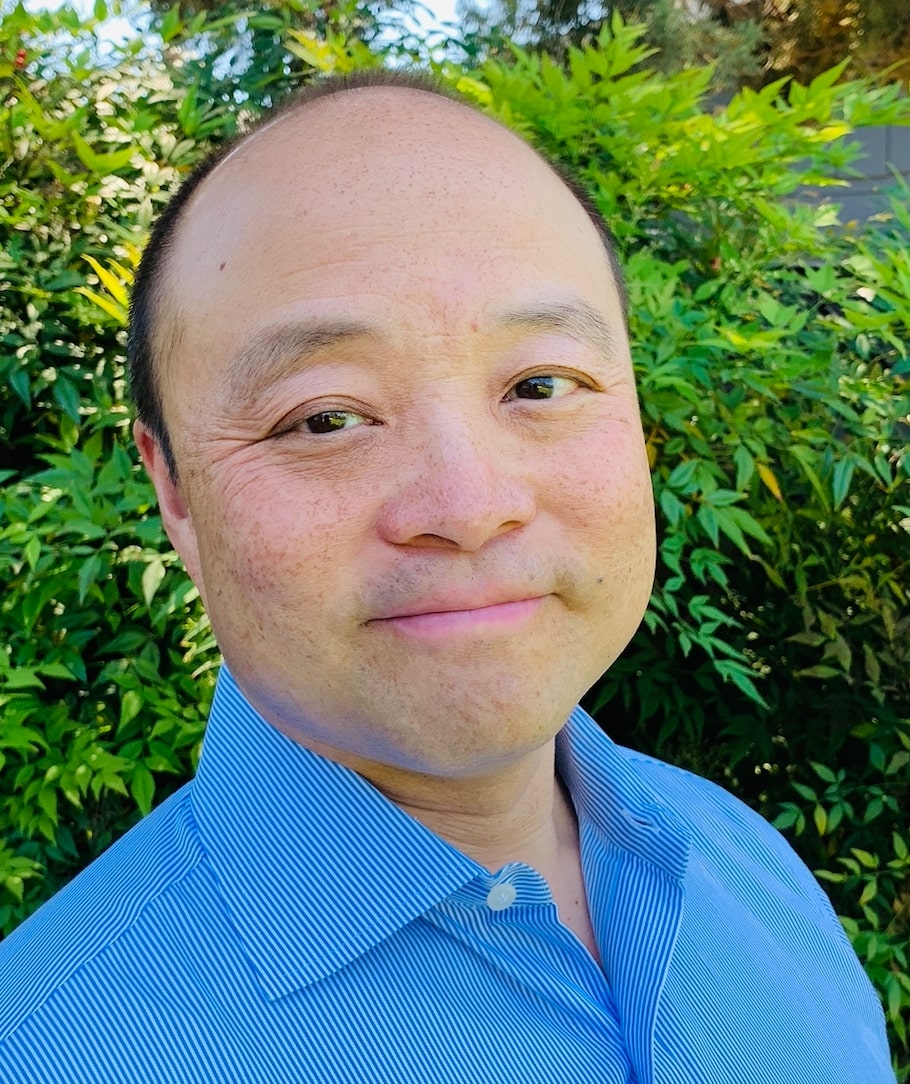
Brian Wang is a Futurist Thought Leader and a popular Science blogger with 1 million readers per month. His blog Nextbigfuture.com is ranked #1 Science News Blog. It covers many disruptive technology and trends including Space, Robotics, Artificial Intelligence, Medicine, Anti-aging Biotechnology, and Nanotechnology.
Known for identifying cutting edge technologies, he is currently a Co-Founder of a startup and fundraiser for high potential early-stage companies. He is the Head of Research for Allocations for deep technology investments and an Angel Investor at Space Angels.
A frequent speaker at corporations, he has been a TEDx speaker, a Singularity University speaker and guest at numerous interviews for radio and podcasts. He is open to public speaking and advising engagements.