Physicist Edward Boyda of St. Mary’s College of California in Moraga and colleagues fed hundreds of NASA satellite images of California into the D-Wave 2X processor, which contains 1152 qubits. The researchers asked the computer to consider dozens of features—hue, saturation, even light reflectance—to determine whether clumps of pixels were trees as opposed to roads, buildings, or rivers. They then told the computer whether its classifications were right or wrong so that the computer could learn from its mistakes, tweaking the formula it uses to determine whether something is a tree.
After it was trained, the D-Wave was 90% accurate in recognizing trees in aerial photographs of Mill Valley, California, the team reports in PLOS ONE. It was only slightly more accurate than a conventional computer would have been at the same problem. But the results demonstrate how scientists can program quantum computers to “look” at and analyze images, and opens up the possibility of using them to solve other complex problems that require heavy data crunching.
The validation error rate of 9% cuts by half the error rate from the best of the weak classifiers on their own. The boosted classifier is compact, relatively robust in generalization, and fast in execution: After feature extraction, a sample datum can be classified by tabulating nine less than / greater than comparisons. By feature expansion, the accuracy can be improved to 92% in validation and 90% on the Mill Valley test set. The performance of the classifier likely could be improved further by incorporating a broader set of weak classifiers, in hopes of better capturing the multivalent dependencies of the data, and by increasing the nonlinearity available to the system as expressed in the weak classifiers. The piecewise-polynomial nonlinearity avail- able to boosted decision stumps will never achieve the complex transformations of the input data space that are possible in a deep neural network, and a multilayer perceptron already fits our training data better than does the boosted classifier. As deep learning frameworks grow in complexity, boosting may prove useful to preselect features to input to such networks. In sum, we were able with some effort to construct a viable classifier of tree cover, despite the restrictions posed by the hardware architecture. Whether this framework proves compel- ling in the long run will depend on the maturation of quantum annealing hardware, the gains to be found in larger ensembles of input metrics, and the relative challenge of training competing frameworks at similar scale.
Nemani says the study lays the groundwork for better climate forecasting. By poring over NASA’s satellite imagery, quantum processors could take a machine learning approach to uncover new patterns in how weather moves across the world over the course of weeks, months, or even years, he says. “Say you’re living in India—you might get an advance notice of a cyclone 6 months ahead of time because we see a pattern of weather in northern Canada.”
Abstract
Quantum annealing is an experimental and potentially breakthrough computational technology for handling hard optimization problems, including problems of computer vision. We present a case study in training a production-scale classifier of tree cover in remote sensing imagery, using early-generation quantum annealing hardware built by D-wave Systems, Inc. Beginning within a known boosting framework, we train decision stumps on texture features and vegetation indices extracted from four-band, one-meter-resolution aerial imagery from the state of California. We then impose a regulated quadratic training objective to select an optimal voting subset from among these stumps. The votes of the subset define the classifier. For optimization, the logical variables in the objective function map to quantum bits in the hardware device, while quadratic couplings encode as the strength of physical interactions between the quantum bits. Hardware design limits the number of couplings between these basic physical entities to five or six. To account for this limitation in mapping large problems to the hardware architecture, we propose a truncation and rescaling of the training objective through a trainable metaparameter. The boosting process on our basic 108- and 508-variable problems, thus constituted, returns classifiers that incorporate a diverse range of color- and texture-based metrics and discriminate tree cover with accuracies as high as 92% in validation and 90% on a test scene encompassing the open space preserves and dense suburban build of Mill Valley, CA
SOURCES- Science, PLOS One
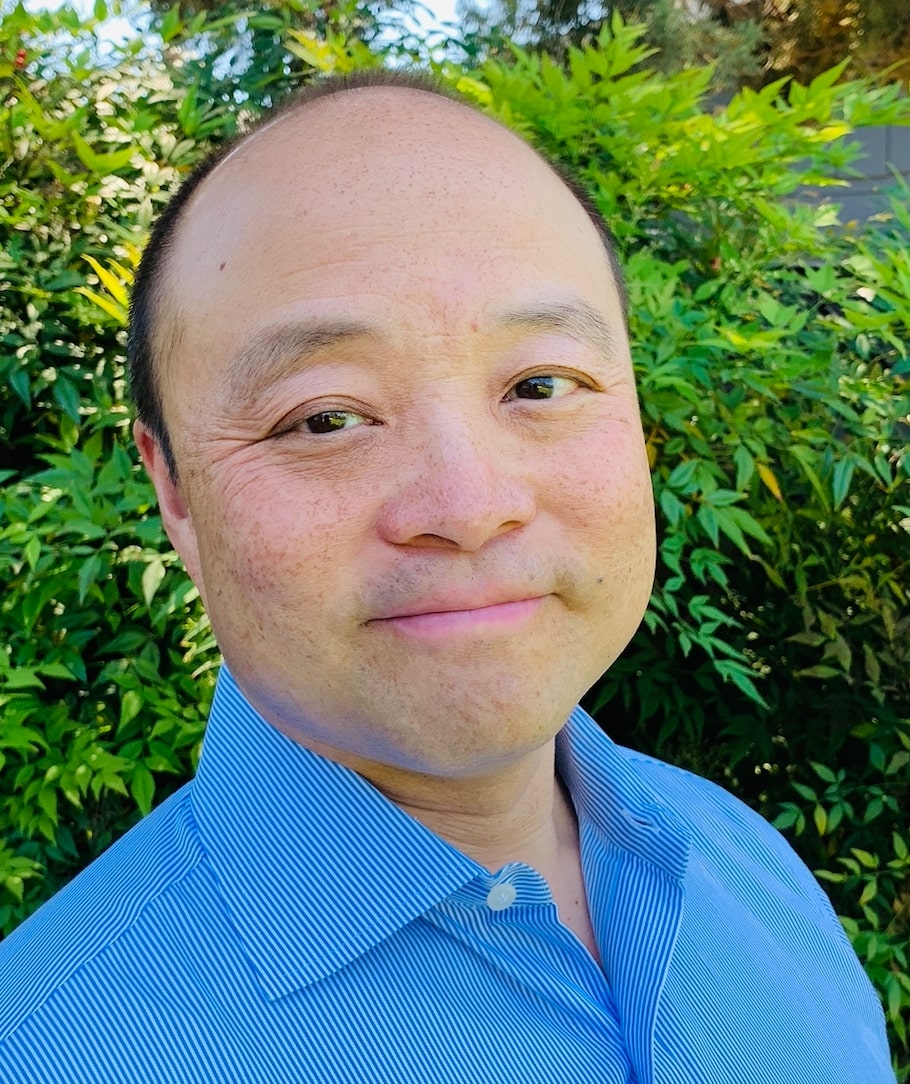
Brian Wang is a Futurist Thought Leader and a popular Science blogger with 1 million readers per month. His blog Nextbigfuture.com is ranked #1 Science News Blog. It covers many disruptive technology and trends including Space, Robotics, Artificial Intelligence, Medicine, Anti-aging Biotechnology, and Nanotechnology.
Known for identifying cutting edge technologies, he is currently a Co-Founder of a startup and fundraiser for high potential early-stage companies. He is the Head of Research for Allocations for deep technology investments and an Angel Investor at Space Angels.
A frequent speaker at corporations, he has been a TEDx speaker, a Singularity University speaker and guest at numerous interviews for radio and podcasts. He is open to public speaking and advising engagements.