Google’s Gemini LLMs (Large Language Model) produced a new math discoveries and solves real-world problems. This is a significant step to AGI. The AI is speeding up progress in math and science.
This is the first time a new discovery has been made for challenging open problems in science or mathematics using LLMs. FunSearch discovered new solutions… its solutions could potentially be slotted into a variety of real-world industrial systems to bring swift benefits… the power of these models can be harnessed not only to produce new mathematical discoveries, but also to reveal potentially impactful solutions to important real-world problems.
An Australian mathematician said the open math problem (best bounds for cap sets) was his favorite open question. Six months ago, Aussie Prof Terry Tao, said would take three years for LLM to reach this level of capability.
AI expert, Alan Thompson has moved up his conservative estimate of AI reaching AGI (Artificial general intelligence from 61% to 64%.
[Published in Nature] Large Language Models (LLMs) have demonstrated tremendous capabilities in solving complex tasks, from quantitative reasoning to understanding natural language. However, LLMs sometimes suffer from confabulations (or hallucinations) which can result in them making plausible but incorrect statements. This hinders the use of current large models in scientific discovery. Here we introduce FunSearch (short for searching in the function space), an evolutionary procedure based on pairing a pre-trained LLM with a systematic evaluator. We demonstrate the effectiveness of this approach to surpass the best known results in important problems, pushing the boundary of existing LLM-based approaches. Applying FunSearch to a central problem in extremal combinatorics — the cap set problem — we discover new constructions of large cap sets going beyond the best known ones, both in finite dimensional and asymptotic cases. This represents the first discoveries made for established open problems using LLMs. We showcase the generality of FunSearch by applying it to an algorithmic problem, online bin packing, finding new heuristics that improve upon widely used baselines. In contrast to most computer search approaches, FunSearch searches for programs that describe how to solve a problem, rather than what the solution is. Beyond being an effective and scalable strategy, discovered programs tend to be more interpretable than raw solutions, enabling feedback loops between domain experts and FunSearch, and the deployment of such programs in real-world applications.
Alan Thompson will be giving about 30% more to his AGI tracker when we have capable humanoid robots with large language model AIs.
Tesla released the Optimus Gen 2 Teslabot with huge advances in capabilities. The fusion of advancing AI and mass produced humanoid robots is very near.
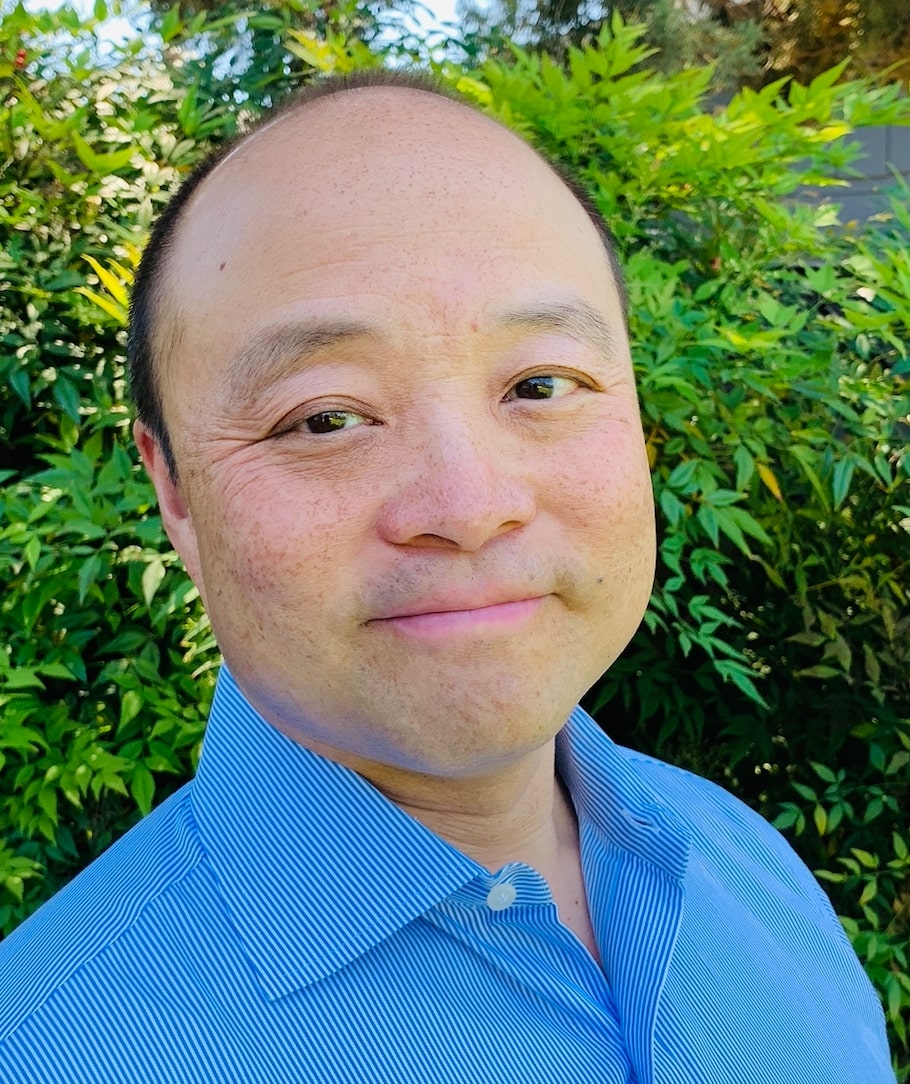
Brian Wang is a Futurist Thought Leader and a popular Science blogger with 1 million readers per month. His blog Nextbigfuture.com is ranked #1 Science News Blog. It covers many disruptive technology and trends including Space, Robotics, Artificial Intelligence, Medicine, Anti-aging Biotechnology, and Nanotechnology.
Known for identifying cutting edge technologies, he is currently a Co-Founder of a startup and fundraiser for high potential early-stage companies. He is the Head of Research for Allocations for deep technology investments and an Angel Investor at Space Angels.
A frequent speaker at corporations, he has been a TEDx speaker, a Singularity University speaker and guest at numerous interviews for radio and podcasts. He is open to public speaking and advising engagements.
An Optimus has yet to perform a penny’s worth of productive labour so let’s not get too excited. They will be spending a significant amount of time in the role of “special needs coop student” with handlers and special supervisors for some time—consuming more productive labour than they produce.
I’m impressed with the speed and quality of the Optimus project. I am very pleased that intelligence simulations are making real world contributions to science and technology. That sure beats making pretty pictures and flashy video. But a singularity is not months away. As we advance our simulated intelligence projects, actual artificial intelligence may be getting closer but we don’t have it yet so talk about AGI is a tad premature.
Every technological singularity (a fuzzy point in history where people living before it can’t properly envisage what things will be like after it) appears in about half the time from its predecessor, than it’s predecessor appeared after its predecessor.
Going back just a bit, we get the Gutenberg press (implementation somewhat delayed by the powers-that-be, or would that be powers-that-were?). Then the industrial revolution (physical automation), electronics, the worldwide web, and now, apparently, cognitive automation (sometimes called by the vague and overused term: AI).
It follows that 15 years after 2023 we are due for the next, then 7.5 years, then 3.75 years, 2 years (rounding) and 1 year. And who knows how many in the year after that.? Surely a new rule would prevent it from getting too crazy, right?
But, yeah, what we have now, not really AI but cognitive evolution. And it appears to be an essential step to getting to something more like AGI or a man-machine hybrid that gets us into the same realm of capabilities, capabilities that are probably necessary for moving beyond radical life extension entirely and into the realm of indefinite lifespans, regardless of what tech or techs are involved in realizing it.
Then what? Self replicating factories and resource gathering facilities? Nano replacement of living cells, in situ? And who knows what other craziness? Fusion? Off-planet resource gathering and manufacturing? Further augmented intelligence? Surrogates? And, of course, a host of potential things we haven’t even considered yet?
I guess a lot of us may just have to wait and see because–do the math–2053 might be a very, very interesting year.
Allright, now just equip them with guns and the US is again the number 1 army in the world for years to come! Haul em over to Europe!
Soldiering is like taking care of the elderly; because there are few people who want to do it we are always hoping for someone (immigrants) or something (robots) to fill the role but that desire blinds us to how hard these jobs are in real life. We’ve had guns on treaded robots and even robotic armoured vehicles for a while now but they aren’t used at significant scales because it is not as cheap or talented as we want them to be.
Another new development in the works:
“The incredible computational power of the human brain can be seen in the way it performs billion-billion mathematical operations per second using only 20 watts of power. DeepSouth achieves similar levels of parallel processing by employing neuromorphic engineering, a design approach that mimics the brain’s functioning.”
See:
https://interestingengineering.com/innovation/human-brain-supercomputer-coming-in-2024
&
https://newatlas.com/computers/human-brain-supercomputer/