Deep Learning and Machine Learning has made breakthroughs in recent years. There is tens of billions of dollars going into development of the new AI.
Google and Deep Mind are recognizing that Deep Learning is not going to reach human cognition. They propose using models of networks to find relations between things to enable computers to generalize more broadly about the world.
Deep learning faces challenges in complex language and scene understanding, reasoning about structured data, transferring learning beyond the training conditions, and learning from small amounts of experience. These challenges demand combinatorial generalization, and so it is perhaps not surprising that an approach which eschews compositionality and explicit structure
struggles to meet them.
A key path forward for modern AI is to commit to combinatorial generalization as a top priority, and we advocate for integrative approaches to realize this goal.
Neural networks that operate on graphs, and structure their computations accordingly, have been developed and explored extensively for more than a decade under the umbrella of “graph neural networks”.
They have a new graph networks framework, which generalizes and extends several lines of work in this area.
They define a class of functions for relational reasoning over graph-structured representations.
They are excited about the potential impacts that graph networks can have, they caution that
these models are only one step forward. Realizing the full potential of graph networks will likely be far more challenging than organizing their behavior under one framework, and indeed, there are a number of unanswered questions regarding the best ways to use graph networks.
Graphs are not able to express everything. Notions like recursion, control flow, and conditional iteration are not straightforward to represent with graphs, and, minimally, require additional assumptions.
Other structural forms will be needed like imitations of computer-based structures, including registers, memory I/O controllers, stacks, queues and others.
Recent advances in AI, propelled by deep learning, have been transformative across many important domains. Despite this, a vast gap between human and machine intelligence remains, especially with respect to efficient, generalizable learning. They argue for making combinatorial generalization a top priority for AI, and advocate for embracing integrative approaches which draw on ideas from human cognition, traditional computer science, standard engineering practice, and modern deep learning.
They explored flexible learning-based approaches which implement strong relational inductive biases to capitalize on explicitly structured representations and computations, and presented a framework called graph networks, which generalize and extend various recent approaches for neural networks applied to graphs. Graph networks are designed to promote building complex architectures using customizable graph-to-graph building blocks, and their relational inductive biases promote combinatorial generalization and improved sample efficiency over other standard machine learning building blocks.
Despite their benefits and potential, however, learnable models which operate on graphs are only a stepping stone on the path toward human-like intelligence. They are optimistic about a number of other relevant, and perhaps underappreciated, research directions, including marrying learning-based approaches with programs, developing model-based approaches with an emphasis on abstraction investing more heavily in meta-learning, and exploring multi-agent learning and interaction as a key catalyst for advanced intelligence. These directions each involve rich notions of entities, relations, and combinatorial generalization, and can potentially benefit, and benefit from, greater interaction with approaches for learning relational reasoning over explicitly structured representations.
Arxiv – Relational inductive biases, deep learning, and graph networks
Artificial intelligence (AI) has undergone a renaissance recently, making major progress in key domains such as vision, language, control, and decision-making. This has been due, in part, to cheap data and cheap compute resources, which have fit the natural strengths of deep learning. However, many defining characteristics of human intelligence, which developed under much different pressures, remain out of reach for current approaches. In particular, generalizing beyond one’s experiences–a hallmark of human intelligence from infancy–remains a formidable challenge for modern AI.
The following is part position paper, part review, and part unification. We argue that combinatorial generalization must be a top priority for AI to achieve human-like abilities, and that structured representations and computations are key to realizing this objective. Just as biology uses nature and nurture cooperatively, we reject the false choice between “hand-engineering” and “end-to-end” learning, and instead advocate for an approach which benefits from their complementary strengths. We explore how using relational inductive biases within deep learning architectures can facilitate learning about entities, relations, and rules for composing them. We present a new building block for the AI toolkit with a strong relational inductive bias–the graph network–which generalizes and extends various approaches for neural networks that operate on graphs, and provides a straightforward interface for manipulating structured knowledge and producing structured behaviors. We discuss how graph networks can support relational reasoning and combinatorial generalization, laying the foundation for more sophisticated, interpretable, and flexible patterns of reasoning. As a companion to this paper, we have released an open-source software library for building graph networks, with demonstrations of how to use them in practice.
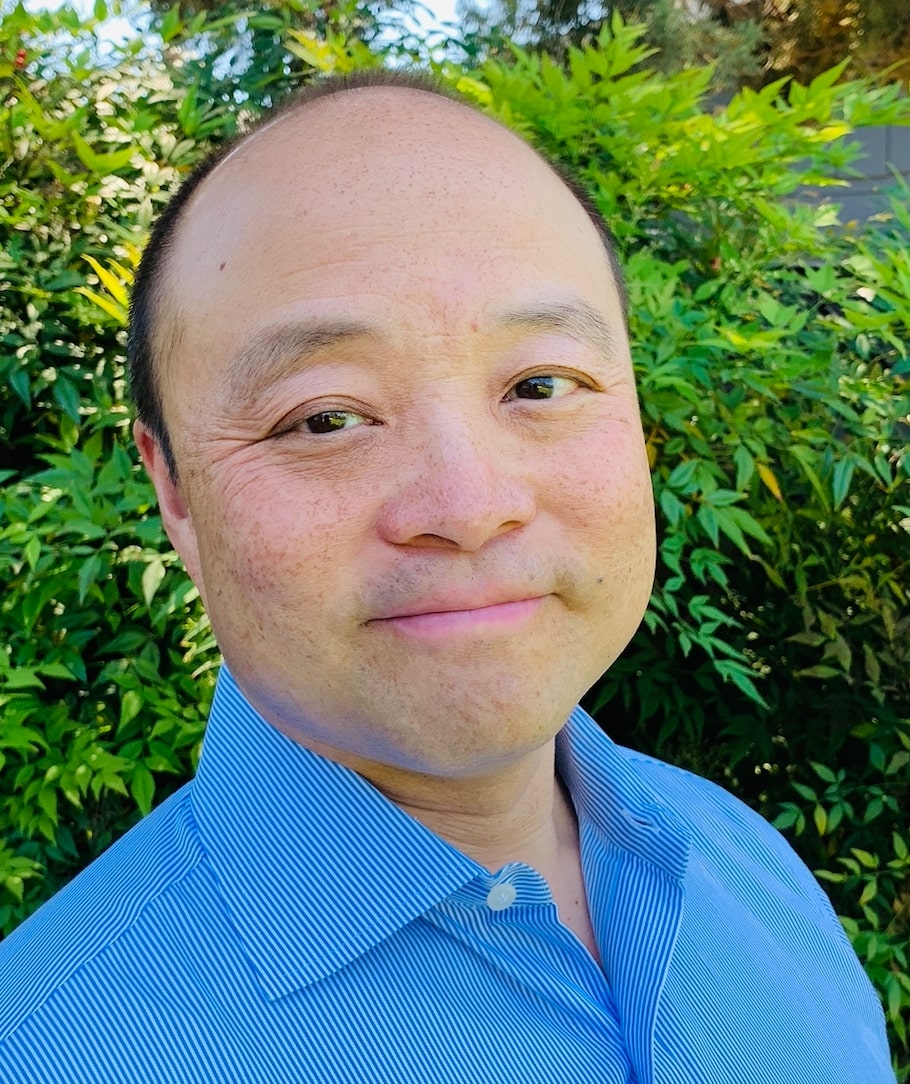
Brian Wang is a Futurist Thought Leader and a popular Science blogger with 1 million readers per month. His blog Nextbigfuture.com is ranked #1 Science News Blog. It covers many disruptive technology and trends including Space, Robotics, Artificial Intelligence, Medicine, Anti-aging Biotechnology, and Nanotechnology.
Known for identifying cutting edge technologies, he is currently a Co-Founder of a startup and fundraiser for high potential early-stage companies. He is the Head of Research for Allocations for deep technology investments and an Angel Investor at Space Angels.
A frequent speaker at corporations, he has been a TEDx speaker, a Singularity University speaker and guest at numerous interviews for radio and podcasts. He is open to public speaking and advising engagements.
… it is perhaps not surprising that an approach which eschews compositionality and explicit structure struggles to meet them” I agree with that. You cannot pretend to build an artificial intelligence without putting intelligence in it. The deep learning technology is nothing more, at the end, than a statistical treatment which never penetrates the core of the problem. It’s obvious that this is very limitating.
… it is perhaps not surprising that an approach which eschews compositionality and explicit structurestruggles to meet them”” I agree with that. You cannot pretend to build an artificial intelligence without putting intelligence in it. The deep learning technology is nothing more”” at the end”” than a statistical treatment which never penetrates the core of the problem. It’s obvious that this is very limitating.”””
Which is why super-intelligence will not be so easy. We are as a whole about as intelligent as possible. The thing that will make us more intelligent is more observations of events, more sensors and cameras and things processing them. As energy becomes cheaper intelligence becomes greater.
Which is why super-intelligence will not be so easy. We are as a whole about as intelligent as possible. The thing that will make us more intelligent is more observations of events more sensors and cameras and things processing them. As energy becomes cheaper intelligence becomes greater.
I believe there is a hard ceiling, the physical constraints of the universe, and our brains are already very efficient. You need to observe astronomical events over time before you can understand physics. The only unlimited aspect is your ability to observe these events, the “eyes”, putting it all together (composing the graph) is not so hard, there is a limited amount of relevant data to connect and your super-intelligent computer would be sitting idle most of the time, not dreaming up limitless information.
http://ai.neocities.org/Skynet.html is next level.
There is not hard ceiling to the intelligence. Does not compute.
I believe there is a hard ceiling the physical constraints of the universe and our brains are already very efficient. You need to observe astronomical events over time before you can understand physics. The only unlimited aspect is your ability to observe these events the eyes””” putting it all together (composing the graph) is not so hard there is a limited amount of relevant data to connect and your super-intelligent computer would be sitting idle most of the time”” not dreaming up limitless information.”””
http://ai.neocities.org/Skynet.html is next level.
There is not hard ceiling to the intelligence. Does not compute.
I suspect human like intelligence will be realized through “humanlike systems” that is neural networks. Since electronic neurons would reach steady state much faster, I wonder if they could be reconfigured continuously to create virtual neural nets with many orders of magnitude more virtual neurons.
I suspect human like intelligence will be realized through humanlike systems”” that is neural networks. Since electronic neurons would reach steady state much faster”””” I wonder if they could be reconfigured continuously to create virtual neural nets with many orders of magnitude more virtual neurons.”””
Wouldn’t it dream up continually better means of collecting information across maximum sample size of the universe? Idling from some kind of diminishing returns dynamic would only happen if that is the structure of the greater universe. Which is a known unknown right now.
Wouldn’t it dream up continually better means of collecting information across maximum sample size of the universe? Idling from some kind of diminishing returns dynamic would only happen if that is the structure of the greater universe. Which is a known unknown right now.
Sure, “it” could continually develop collectors, but see you’ve already determined “it” exists at all. What you’re actually imagining is an uber-powerful version of yourself, but no such thing exists and never will. It would be the science project of some other human being to build that sort of thing. What I believe is AI already exists in the form of the internet. It networked all of the eyes together and now what you see is only marginally better than what came before. Information travels around the world at the speed of light. The only thing left to do is make the cognition happen quicker, broaden the established data set and record trivial minutia, but we are still living in a bubble and you can count the number of photon events happening around us. There are only a relatively tiny number of particles to see at the quantum scale, we could observe that some more, but this idea that there is a way to create an explosion of knowledge and know everything there is to know in one big blast is what I argue against. If I were “it” I would definitely expand the collectors, but only to a certain point that it made sense to. Sending probes outward is a more worthwhile endeavor, I think. Consolidate the information channels, big powerful beams of information transmitted between stars.
Sure it”” could continually develop collectors”””” but see you’ve already determined “”””it”””” exists at all. What you’re actually imagining is an uber-powerful version of yourself”” but no such thing exists and never will. It would be the science project of some other human being to build that sort of thing. What I believe is AI already exists in the form of the internet. It networked all of the eyes together and now what you see is only marginally better than what came before.Information travels around the world at the speed of light. The only thing left to do is make the cognition happen quicker broaden the established data set and record trivial minutia but we are still living in a bubble and you can count the number of photon events happening around us. There are only a relatively tiny number of particles to see at the quantum scale we could observe that some more”” but this idea that there is a way to create an explosion of knowledge and know everything there is to know in one big blast is what I argue against.If I were “”””it”””” I would definitely expand the collectors”” but only to a certain point that it made sense to. Sending probes outward is a more worthwhile endeavor I think. Consolidate the information channels”” big powerful beams of information transmitted between stars.”””
I’m not sure I understand the arguments, so I’m trying to parse piecewise: > Why is what I suggested not basic consequence of how sampling works? > Is this idea falsifiable ? > Why is it certain that there’s nothing in between those two extremes? Or I misunderstood. > Yes that’s what I meant to refer to by “increasing sample size”. It’s a big universe; and a very long time to heat death. It doesn’t seem (to me) that we’ve got enough of a grasp on the laws of nature to assert we’re anywhere near the point of diminishing returns, even in a thought experiment. I would argue we can’t start to make that kind of assertion until we’ve got a majority of the observable universe under our observation. And then the scope of our assertions would exclude the unobservable universe. Either way it doesn’t seem to me that we’re ready to take for granted that it doesn’t get any better, in terms of computational power and data gathering to feed that computation, than c.2018 internet/humans symbiote.
I’m not sure I understand the arguments so I’m trying to parse piecewise:Yes that’s what I meant to refer to by increasing sample size””. It’s a big universe; and a very long time to heat death. It doesn’t seem (to me) that we’ve got enough of a grasp on the laws of nature to assert we’re anywhere near the point of diminishing returns”” even in a thought experiment. I would argue we can’t start to make that kind of assertion until we’ve got a majority of the observable universe under our observation. And then the scope of our assertions would exclude the unobservable universe. Either way it doesn’t seem to me that we’re ready to take for granted that it doesn’t get any better in terms of computational power and data gathering to feed that computation”” than c.2018 internet/humans symbiote.”””
I’m not sure I understand the arguments, so I’m trying to parse piecewise: > Why is what I suggested not basic consequence of how sampling works? > Is this idea falsifiable ? > Why is it certain that there’s nothing in between those two extremes? Or I misunderstood. > Yes that’s what I meant to refer to by “increasing sample size”. It’s a big universe; and a very long time to heat death. It doesn’t seem (to me) that we’ve got enough of a grasp on the laws of nature to assert we’re anywhere near the point of diminishing returns, even in a thought experiment. I would argue we can’t start to make that kind of assertion until we’ve got a majority of the observable universe under our observation. And then the scope of our assertions would exclude the unobservable universe. Either way it doesn’t seem to me that we’re ready to take for granted that it doesn’t get any better, in terms of computational power and data gathering to feed that computation, than c.2018 internet/humans symbiote.
I’m not sure I understand the arguments so I’m trying to parse piecewise:Yes that’s what I meant to refer to by increasing sample size””. It’s a big universe; and a very long time to heat death. It doesn’t seem (to me) that we’ve got enough of a grasp on the laws of nature to assert we’re anywhere near the point of diminishing returns”” even in a thought experiment. I would argue we can’t start to make that kind of assertion until we’ve got a majority of the observable universe under our observation. And then the scope of our assertions would exclude the unobservable universe. Either way it doesn’t seem to me that we’re ready to take for granted that it doesn’t get any better in terms of computational power and data gathering to feed that computation”” than c.2018 internet/humans symbiote.”””
Sure, “it” could continually develop collectors, but see you’ve already determined “it” exists at all. What you’re actually imagining is an uber-powerful version of yourself, but no such thing exists and never will. It would be the science project of some other human being to build that sort of thing. What I believe is AI already exists in the form of the internet. It networked all of the eyes together and now what you see is only marginally better than what came before. Information travels around the world at the speed of light. The only thing left to do is make the cognition happen quicker, broaden the established data set and record trivial minutia, but we are still living in a bubble and you can count the number of photon events happening around us. There are only a relatively tiny number of particles to see at the quantum scale, we could observe that some more, but this idea that there is a way to create an explosion of knowledge and know everything there is to know in one big blast is what I argue against. If I were “it” I would definitely expand the collectors, but only to a certain point that it made sense to. Sending probes outward is a more worthwhile endeavor, I think. Consolidate the information channels, big powerful beams of information transmitted between stars.
Sure it”” could continually develop collectors”””” but see you’ve already determined “”””it”””” exists at all. What you’re actually imagining is an uber-powerful version of yourself”” but no such thing exists and never will. It would be the science project of some other human being to build that sort of thing. What I believe is AI already exists in the form of the internet. It networked all of the eyes together and now what you see is only marginally better than what came before.Information travels around the world at the speed of light. The only thing left to do is make the cognition happen quicker broaden the established data set and record trivial minutia but we are still living in a bubble and you can count the number of photon events happening around us. There are only a relatively tiny number of particles to see at the quantum scale we could observe that some more”” but this idea that there is a way to create an explosion of knowledge and know everything there is to know in one big blast is what I argue against.If I were “”””it”””” I would definitely expand the collectors”” but only to a certain point that it made sense to. Sending probes outward is a more worthwhile endeavor I think. Consolidate the information channels”” big powerful beams of information transmitted between stars.”””
I’m not sure I understand the arguments, so I’m trying to parse piecewise:
<< What you're actually imagining is an uber-powerful version of yourself,>>
Why is what I suggested not basic consequence of how sampling works?
<< What I believe is AI already exists in the form of the internet. It networked all of the eyes together and now what you see is only marginally better than what came before. >>
Is this idea falsifiable ?
<< broaden the established data set [...] but this idea that there is a way to create an explosion of knowledge and know everything there is to know in one big blast >>
Why is it certain that there’s nothing in between those two extremes? Or I misunderstood.
<< Sending probes outward is a more worthwhile endeavor, I think. >>
Yes that’s what I meant to refer to by “increasing sample size”. It’s a big universe; and a very long time to heat death. It doesn’t seem (to me) that we’ve got enough of a grasp on the laws of nature to assert we’re anywhere near the point of diminishing returns, even in a thought experiment. I would argue we can’t start to make that kind of assertion until we’ve got a majority of the observable universe under our observation.
And then the scope of our assertions would exclude the unobservable universe.
Either way it doesn’t seem to me that we’re ready to take for granted that it doesn’t get any better, in terms of computational power and data gathering to feed that computation, than c.2018 internet/humans symbiote.
Wouldn’t it dream up continually better means of collecting information across maximum sample size of the universe? Idling from some kind of diminishing returns dynamic would only happen if that is the structure of the greater universe. Which is a known unknown right now.
Wouldn’t it dream up continually better means of collecting information across maximum sample size of the universe? Idling from some kind of diminishing returns dynamic would only happen if that is the structure of the greater universe. Which is a known unknown right now.
Sure, “it” could continually develop collectors, but see you’ve already determined “it” exists at all. What you’re actually imagining is an uber-powerful version of yourself, but no such thing exists and never will. It would be the science project of some other human being to build that sort of thing. What I believe is AI already exists in the form of the internet. It networked all of the eyes together and now what you see is only marginally better than what came before.
Information travels around the world at the speed of light. The only thing left to do is make the cognition happen quicker, broaden the established data set and record trivial minutia, but we are still living in a bubble and you can count the number of photon events happening around us. There are only a relatively tiny number of particles to see at the quantum scale, we could observe that some more, but this idea that there is a way to create an explosion of knowledge and know everything there is to know in one big blast is what I argue against.
If I were “it” I would definitely expand the collectors, but only to a certain point that it made sense to. Sending probes outward is a more worthwhile endeavor, I think. Consolidate the information channels, big powerful beams of information transmitted between stars.
Wouldn’t it dream up continually better means of collecting information across maximum sample size of the universe? Idling from some kind of diminishing returns dynamic would only happen if that is the structure of the greater universe. Which is a known unknown right now.
I suspect human like intelligence will be realized through “humanlike systems” that is neural networks. Since electronic neurons would reach steady state much faster, I wonder if they could be reconfigured continuously to create virtual neural nets with many orders of magnitude more virtual neurons.
I suspect human like intelligence will be realized through humanlike systems”” that is neural networks. Since electronic neurons would reach steady state much faster”””” I wonder if they could be reconfigured continuously to create virtual neural nets with many orders of magnitude more virtual neurons.”””
I believe there is a hard ceiling, the physical constraints of the universe, and our brains are already very efficient. You need to observe astronomical events over time before you can understand physics. The only unlimited aspect is your ability to observe these events, the “eyes”, putting it all together (composing the graph) is not so hard, there is a limited amount of relevant data to connect and your super-intelligent computer would be sitting idle most of the time, not dreaming up limitless information.
I believe there is a hard ceiling the physical constraints of the universe and our brains are already very efficient. You need to observe astronomical events over time before you can understand physics. The only unlimited aspect is your ability to observe these events the eyes””” putting it all together (composing the graph) is not so hard there is a limited amount of relevant data to connect and your super-intelligent computer would be sitting idle most of the time”” not dreaming up limitless information.”””
http://ai.neocities.org/Skynet.html is next level.
http://ai.neocities.org/Skynet.html is next level.
I suspect human like intelligence will be realized through “humanlike systems” that is neural networks. Since electronic neurons would reach steady state much faster, I wonder if they could be reconfigured continuously to create virtual neural nets with many orders of magnitude more virtual neurons.
There is not hard ceiling to the intelligence. Does not compute.
There is not hard ceiling to the intelligence. Does not compute.
Which is why super-intelligence will not be so easy. We are as a whole about as intelligent as possible. The thing that will make us more intelligent is more observations of events, more sensors and cameras and things processing them. As energy becomes cheaper intelligence becomes greater.
Which is why super-intelligence will not be so easy. We are as a whole about as intelligent as possible. The thing that will make us more intelligent is more observations of events more sensors and cameras and things processing them. As energy becomes cheaper intelligence becomes greater.
I believe there is a hard ceiling, the physical constraints of the universe, and our brains are already very efficient. You need to observe astronomical events over time before you can understand physics. The only unlimited aspect is your ability to observe these events, the “eyes”, putting it all together (composing the graph) is not so hard, there is a limited amount of relevant data to connect and your super-intelligent computer would be sitting idle most of the time, not dreaming up limitless information.
http://ai.neocities.org/Skynet.html is next level.
… it is perhaps not surprising that an approach which eschews compositionality and explicit structure struggles to meet them” I agree with that. You cannot pretend to build an artificial intelligence without putting intelligence in it. The deep learning technology is nothing more, at the end, than a statistical treatment which never penetrates the core of the problem. It’s obvious that this is very limitating.
… it is perhaps not surprising that an approach which eschews compositionality and explicit structurestruggles to meet them”” I agree with that. You cannot pretend to build an artificial intelligence without putting intelligence in it. The deep learning technology is nothing more”” at the end”” than a statistical treatment which never penetrates the core of the problem. It’s obvious that this is very limitating.”””
There is not hard ceiling to the intelligence. Does not compute.
Which is why super-intelligence will not be so easy. We are as a whole about as intelligent as possible. The thing that will make us more intelligent is more observations of events, more sensors and cameras and things processing them. As energy becomes cheaper intelligence becomes greater.
“… it is perhaps not surprising that an approach which eschews compositionality and explicit structure
struggles to meet them” I agree with that. You cannot pretend to build an artificial intelligence without putting intelligence in it. The deep learning technology is nothing more, at the end, than a statistical treatment which never penetrates the core of the problem. It’s obvious that this is very limitating.