What are the predictors of successful life outcomes ?
Outcomes being defined as healthier and more productive
It is not claimed skills like C, C++. Those are actually negative predictors.
Mindset and personality (grit and personality)
Basic intelligence
Internal motivation – becoming a fanatic about what you do.
Helping people reach their full potential could provide a 5-10% boost in GDP.
Vivienne developed the Muse system and one month it will be out of beta.
A similar talk was given in 2015.
Currently, a text-based system is appropriate. Today they use SMS – although it’s a hugely regressive system that is expensive. To reach 1 million kids each year it costs about $5 million per year. To reach that same number of kids using WhatsApp or a similar platform costs about $40 per year.
Prior systems have shown that just the daily reminder to a parent to perform a specific activity with their child is beneficial – in our case, with all this data and analysis, our system can ask the one question that we predict will be the most valuable thing for your child on that day.
In terms of our predictive models, think of this more in an actuarial sense: on average, given everything we know about a particular kid, he is more likely to live 5 years longer, she is more likely to go 2 years further in their education, etc. The important thing, our goal, is for none of it to come true, no matter how positive the prediction is. We believe it can always be more. Everyone can be amazing. This may sound like a line, but quite frankly if you don’t believe that you shouldn’t be an educator.
We did the following analysis in the United States: if we simply took the kind of things we do with MUSE, and were able to scale that to every kid in the USA (50 million) and had started this 25 years ago, what would the net effect on the US economy be? We didn’t do broad strokes and wishful thinking – we modeled based on actual research (do this little proactive change when kids are young, then observe that in 25 years they are earning 25% more and have better health outcomes, etc.). We took that actual research, and modeled it out, region by region in the USA; demographics, everything. We found that after 25 years we would have added somewhere between $1.3-1.8 trillion to the US economy.
MUSE is comprised of eight completely independent highly sophisticated machine learning systems, along with integration, data, analytics and interface layers. These systems are analyzing the images, the audio, producing the questions, making the predictions. We use what’s termed a ‘deep reinforcement learning model’ – a very similar concept, at least at a high level, to Google’s “Alpha Go” AI system. This type of system can learn to play highly complex games (Go, video games, etc.) – a fundamentally different type of intelligence than IBM’s older chess-playing programs. This new type of AI actually learns how to play the game itself, as opposed to selecting procedures that have already been programmed into it.
Vivienne Ming is a theoretical neuroscientist, technologist and entrepreneur. She co-founded Socos, where machine learning and cognitive neuroscience combine to maximize students’ life outcomes. Vivienne is also a visiting scholar at UC Berkeley’s Redwood Center for Theoretical Neuroscience, where she pursues her research in neuroprosthetics. In her free time, Vivienne has developed a predictive model of diabetes to better manage the glucose levels of her diabetic son and systems to predict manic episodes in bipolar suffers. She sits on the boards of StartOut, The Palm Center, Emozia, Engender, and Genderis Inc., and is a Chief Science Advisor to Cornerstone Capital, Platypus Institute, Shiftgig, and Bayes Impact. Dr. Ming also speaks frequently on issues of LGBT inclusion and gender in technology. Vivienne lives in Berkeley, CA, with her wife (and co-founder) and their two children.
Here is a 22 page paper from 2014
SOURCES -Youtube
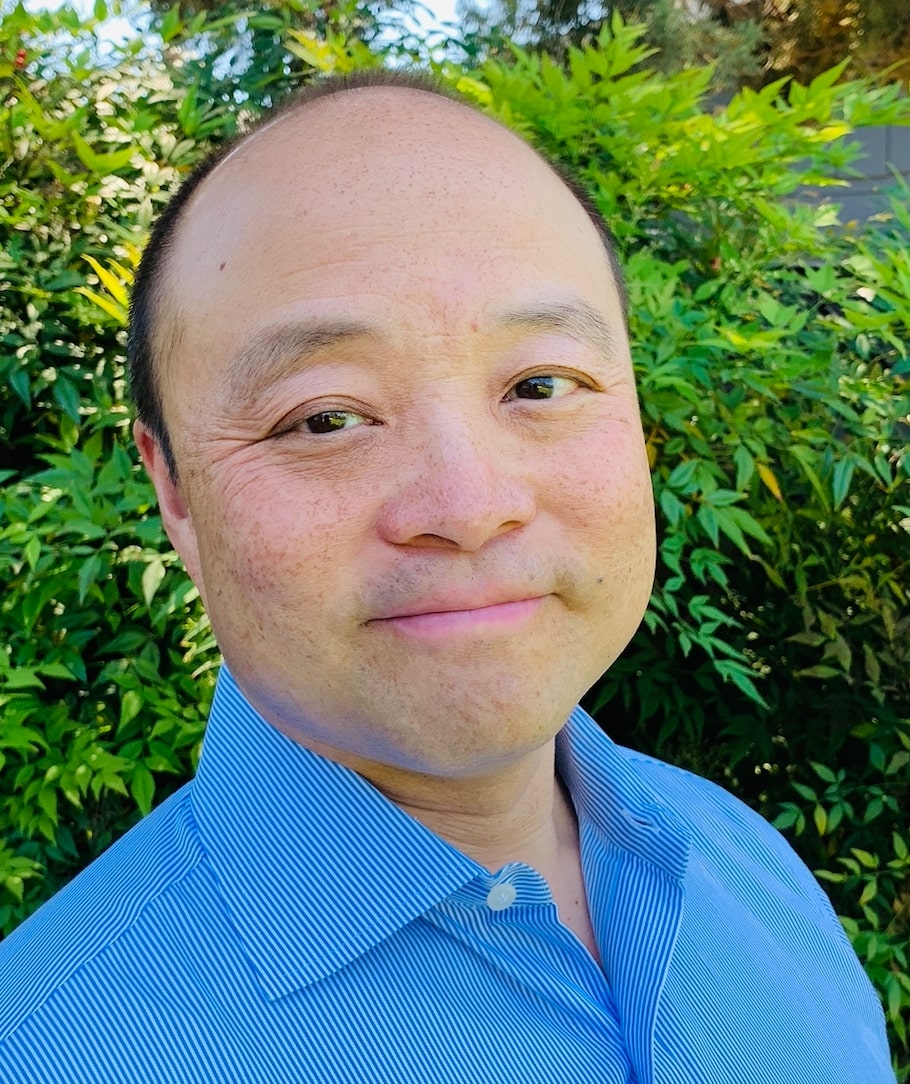
Brian Wang is a Futurist Thought Leader and a popular Science blogger with 1 million readers per month. His blog Nextbigfuture.com is ranked #1 Science News Blog. It covers many disruptive technology and trends including Space, Robotics, Artificial Intelligence, Medicine, Anti-aging Biotechnology, and Nanotechnology.
Known for identifying cutting edge technologies, he is currently a Co-Founder of a startup and fundraiser for high potential early-stage companies. He is the Head of Research for Allocations for deep technology investments and an Angel Investor at Space Angels.
A frequent speaker at corporations, he has been a TEDx speaker, a Singularity University speaker and guest at numerous interviews for radio and podcasts. He is open to public speaking and advising engagements.