Argonne researchers use genetic algorithms to find better superconductors. Certain patterns of defects composed of metallic inclusions were found to maximize the critical current.
researchers developed a computer algorithm that treated each defect like a biological gene. Different combinations of defects yielded superconductors able to carry different amounts of current. Once the algorithm identified a particularly advantageous set of defects, it re-initialized with that set of defects as a “seed,” from which new combinations of defects would emerge.
“Each run of the simulation is equivalent to the formation of a new generation of defects that the algorithm seeks to optimize,” said Argonne distinguished fellow and senior materials scientist Wai-Kwong Kwok, an author of the study. “Over time, the defect structures become progressively refined, as we intentionally select for defect structures that will allow for materials with the highest critical current.”
The reason defects form such an essential part of a superconductor lies in their ability to trap and anchor magnetic vortices that form in the presence of a magnetic field. These vortices can move freely within a pure superconducting material when a current is applied. When they do so, they start to generate a resistance, negating the superconducting effect. Keeping vortices pinned, while still allowing current to travel through the material, represents a holy grail for scientists seeking to find ways to transmit electricity without loss in applied superconductors.
To find the right combination of defects to arrest the motion of the vortices, the researchers initialized their algorithm with defects of random shape and size. While the researchers knew this would be far from the optimal setup, it gave the model a set of neutral initial conditions from which to work. As the researchers ran through successive generations of the model, they saw the initial defects transform into a columnar shape and ultimately a periodic arrangement of planar defects.
“When people think of targeted evolution, they might think of people who breed dogs or horses,” said Argonne materials scientist Andreas Glatz, the corresponding author of the study. “Ours is an example of materials by design, where the computer learns from prior generations the best possible arrangement of defects.”
One potential drawback to the process of artificial defect selection lies in the fact that certain defect patterns can become entrenched in the model, leading to a kind of calcification of the genetic data. “In a certain sense, you can kind of think of it like inbreeding,” Kwok said. “Conserving most information in our defect ‘gene pool’ between generations has both benefits and limitations as it does not allow for drastic systemwide transformations. However, our digital ‘evolution’ can be repeated with different initial seeds to avoid these problems.”
In order to run their model, the researchers required high-performance computing facilities at Argonne and Oak Ridge National Laboratory. The Argonne Leadership Computing Facility and Oak Ridge Leadership Computing Facility are both DOE Office of Science User Facilities.
PNAS – Targeted evolution of pinning landscapes for large superconducting critical currents
Significance
Lossless transport is the Holy Grail of energy science in general and superconductivity research in particular. The main obstacle is the dissipative motion of Abrikosov vortices, which can be reduced or eliminated by pinning at nonsuperconducting defects. Pinning effectiveness nontrivially depends on various factors such as the shape, concentration, and spatial distribution of defects, rendering the optimization of the vortex pinning landscape highly difficult. Here, we use concepts from biological evolution to develop an efficient strategy for vortex pinning improvement. We replace natural selection with targeted selection, where only pinning configurations with better vortex immobilization survive. In combination with high-performance numerical algorithms, it allows us to dynamically evolve the defect landscape into the best possible pinning configuration with maximal lossless current.
Abstract
The ability of type II superconductors to carry large amounts of current at high magnetic fields is a key requirement for future design innovations in high-field magnets for accelerators and compact fusion reactors, and largely depends on the vortex pinning landscape comprised of material defects. The complex interaction of vortices with defects that can be grown chemically, e.g., self-assembled nanoparticles and nanorods, or introduced by postsynthesis particle irradiation precludes a priori prediction of the critical current and can result in highly nontrivial effects on the critical current. Here, we borrow concepts from biological evolution to create a vortex pinning genome based on a genetic algorithm, naturally evolving the pinning landscape to accommodate vortex pinning and determine the best possible configuration of inclusions for two different scenarios: a natural evolution process initiating from a pristine system and one starting with preexisting defects to demonstrate the potential for a postprocessing approach to enhance critical currents. Furthermore, the presented approach is even more general and can be adapted to address various other targeted material optimization problems.
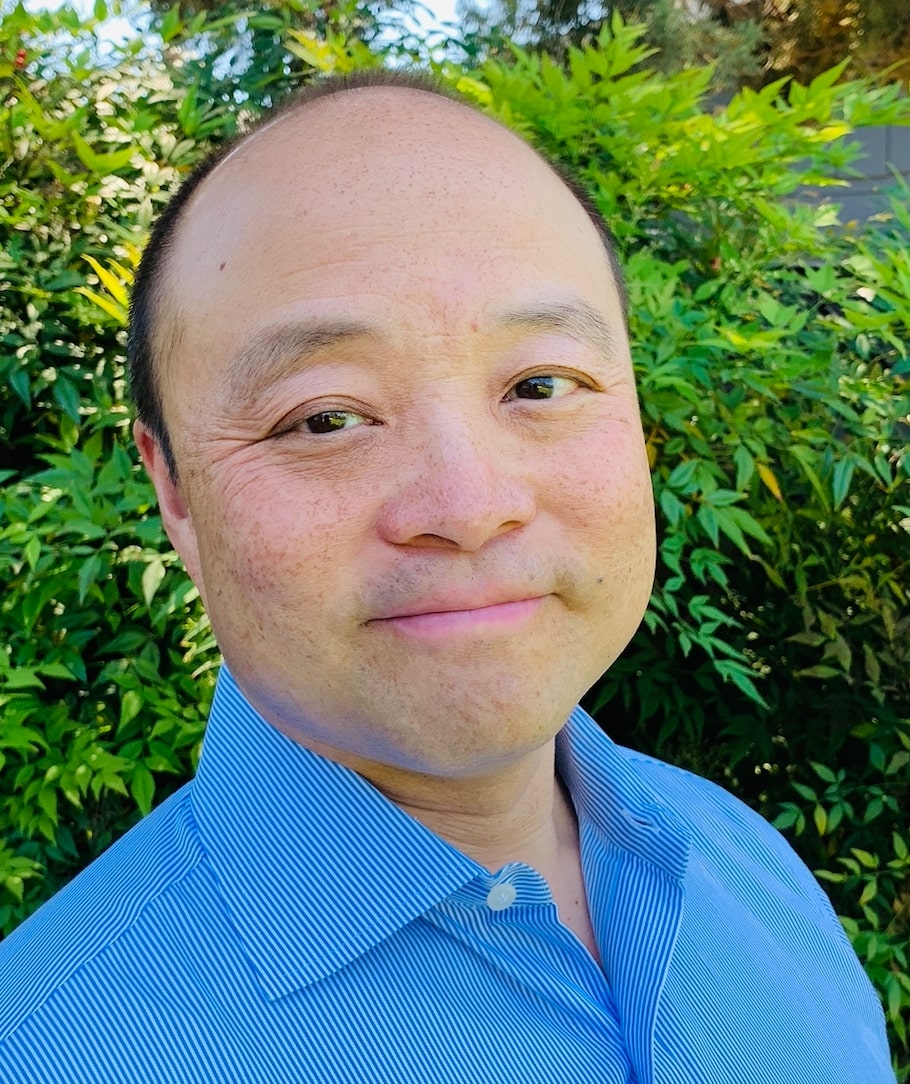
Brian Wang is a Futurist Thought Leader and a popular Science blogger with 1 million readers per month. His blog Nextbigfuture.com is ranked #1 Science News Blog. It covers many disruptive technology and trends including Space, Robotics, Artificial Intelligence, Medicine, Anti-aging Biotechnology, and Nanotechnology.
Known for identifying cutting edge technologies, he is currently a Co-Founder of a startup and fundraiser for high potential early-stage companies. He is the Head of Research for Allocations for deep technology investments and an Angel Investor at Space Angels.
A frequent speaker at corporations, he has been a TEDx speaker, a Singularity University speaker and guest at numerous interviews for radio and podcasts. He is open to public speaking and advising engagements.
PUBLIC DISCLOSURE:
You might realize the above configuration is as a spiral merry-go-round…conic vectors…
Materials surfaced contingencies, nature itself is a symbol of the assembly.
Anent the axial is di-symmetry between DNA and an individual’s brain waves
at the center of the top of the surface. (A Prism Gate) the human body’s interior
is (soft) heat and moisture…what are the string configurations as correlation, a quantum
computer chip will encode a human body’s bio-chemistry signals (systems components composites) how are theses strings generated as non-physical, non-material causality?
Composition configurations arcs in waves (primary, secondary research)
Bertrand’s Paradox, quantum computers represent potential state of the art Super Intelligent Cognitive Machines…sicm+
SIGMA + encoder podcast + quantum intelligence
cation exchange feasibility
https://www.edge.org/conversation/george_dyson-nsa-the-decision-problem
https://www.youtube.com/watch?v=8nt3edWLgIg
crystal cosmic lattice
http://fafnir.phyast.pitt.edu/particles/conuni5.html